When a fast-casual restaurant chain started to see stagnating sales, the company’s CEO came up with a solution: adding new, higher-quality menu items.
To validate his thinking, he informally talked a few dozen diners at different locations, asking them how they would feel about more and higher-quality menu items. After getting enthusiastic responses from those customers, he went ahead with his plan. Sales fell further.
So what went wrong? Statistical analysis showed that what created the most value for customers was a fast dining experience with a short wait time, a simple menu with a few items, ample parking and a bill under $12 per meal. These benefits created the biggest lift in customer value for this restaurant.
Higher quality and an expanded menu did not create any lift in customer value. Where the company’s CEO went wrong was by relying on salience instead of lift potential.
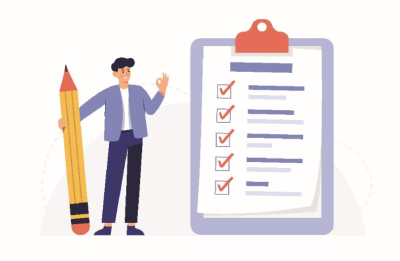
Salience refers to factors that are top-of-mind and easy to recall, which become prominent and are then incorrectly prioritized. A classic example is a 1979 study that surveyed people about their perceived risk of dying from causes like drowning, murder or cancer. The study’s authors found that people thought they were more likely to die from causes that were mentioned more often in their local news, such as murder, when in fact they were at much greater risk of dying from common but less prominent causes such as cancer.
The CEO made food quality and expanded offerings salient to himself by talking about them to a small group of customers. It was an easy way for him to feel good about his efforts.
Relying on salience is easy and convenient, but it’s also the curse of decision-making. It simply reinforces executives’ prior beliefs rather than diagnosing the true lift potential of relationships. Imagine if a doctor saw a patient with stomach pain and recommended an appendectomy because a patient she’d seen the day before needed one. Or if the doctor asked the patient to recommend the treatment himself. Patient outcomes would likely falter and the doctor would go out of business (and perhaps lose her medical license).
Thankfully, doctors don’t operate this way. They rely on the statistically measurable relationship between critical inputs and outputs for decision-making. So should senior executives and CEOs.
Informal customer conversations draw primarily on gut feelings, hunches and top-of-mind ideas, and as such, aren’t reliable indicators of true customer value. Like all of us, customers often tailor their responses to the audience they’re addressing. So a company’s vice president of service might speak with a customer who says they love the service, while the same customer might tell an HR executive they love the employees and then go on to tell the VP of sales that they would like lower prices. These on-the-spot responses typically have no significant impact on or statistical correlation with customer value, which ultimately drives sales and profits.
To craft a successful strategy, executives need to use a systematic, statistical process that starts with choosing a clear outcome or output, such as customer value or employee retention. The next step is to measure inputs that drive that output, and then quantitatively correlate each input to the output. Only those inputs that drive the desired output should be included in the company’s strategy.
Take, for example, a nursing home that attempted to craft a strategy for decreasing employee turnover. Relying on casual conversations with a few dozen employees, executives assumed higher pay would increase retention. They were wrong.
When they statistically correlated multiple inputs — higher pay, health benefits, supervisor respect, promotion opportunities and paid vacation — with retention, they realized their intuitive leaps had been incorrect. Only health insurance and promotions were correlated with increased employee retention. Higher pay had no effect.
Using statistical analysis to unearth the lift potential of different inputs and drive strategy through analytics requires humility on the part of senior executives. The nursing home executives were able to look past their own assumptions and learn from this type of statistical analysis, recognizing the limits of salience-driven thinking and deferring to algorithms that could better predict the inputs of turnover than they could.
Doctors understand this as well. To treat their patients, they rely on data from groups like the Food and Drug Administration or the National Institutes of Health, which run clinical trials and rely on data, statistics and an infrastructure of knowledge.
Unfortunately, many senior executives lack humility when it comes to strategic planning. They equate decades of salience-laden thinking with a deep understanding of correlations between inputs and strategic outputs. They might think, “I’ve been in this industry long enough to know what works,” or “Since this worked then, it will work now as well.” But more often than not, relying on salience-laden intuition alone will not achieve the desired outcome.
References:
- Based on my earlier article: https://houston.innovationmap.com/rice-business-vikas-mittal-intuition-2657151703.html